How AI is Changing Cancer Medicine
The Quiet Revolution
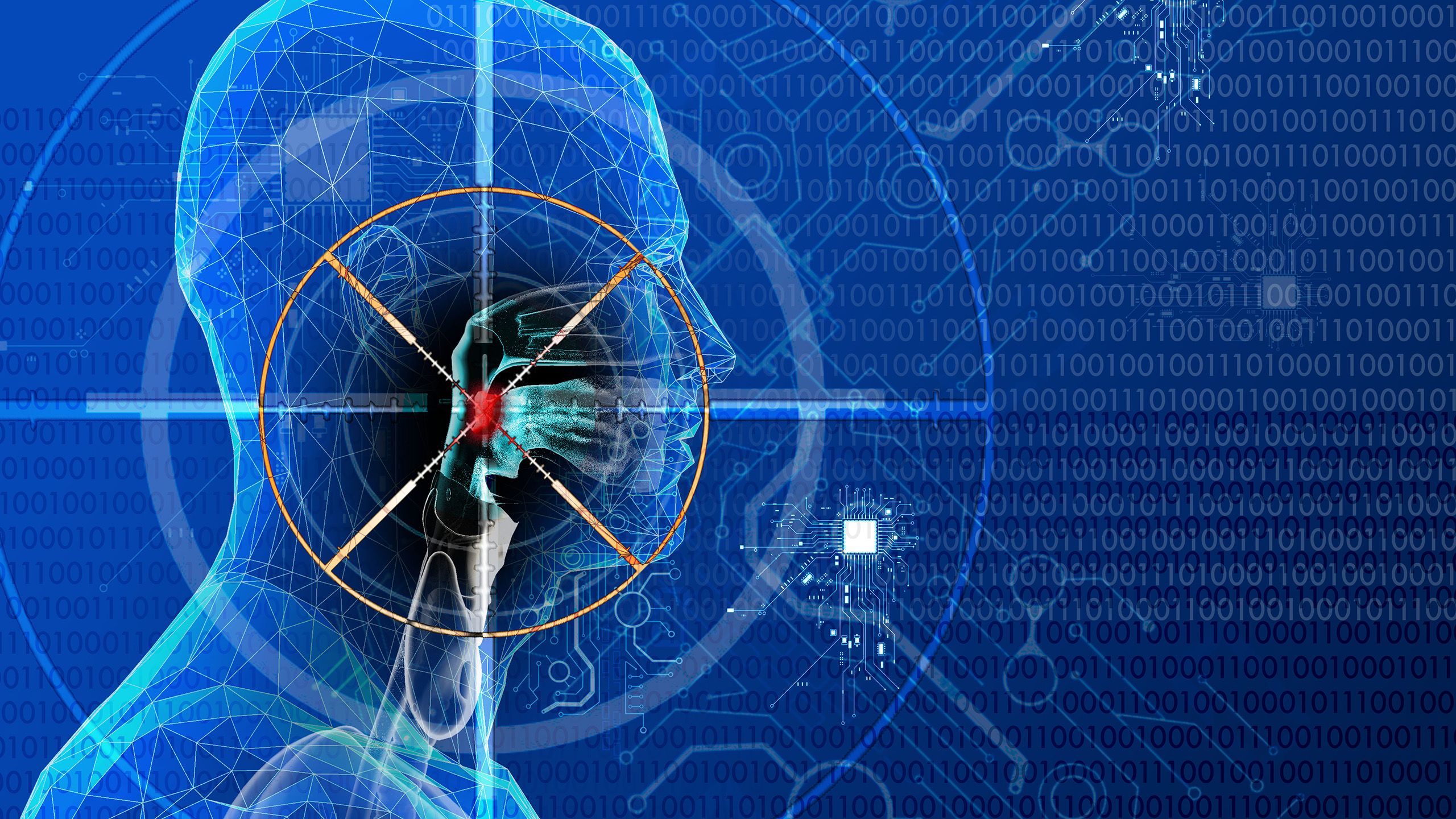
Nabil F. Saba, MD
Nabil F. Saba, MD
Nearly a decade ago, Winship Cancer Institute head and neck cancer specialist and medical oncologist Nabil Saba, MD, helped diagnose a patient with what looked like easily treatable tonsil cancer. “We told him, ‘You have a 95% chance or higher to get cured from this,’” Saba recalls.
Sometimes in cancer, though, the statistics don’t pan out in a patient’s favor, even an athlete in his early 30s. “Despite the clinical picture,” says Saba, “he had recurrent disease that was metastatic and that was not at the time responsive to treatment.”
Saba, The Lynne and Howard Halpern Chair in Head and Neck Cancer Research at Winship and professor and vice chair for quality and safety in the Department of Hematology and Medical Oncology at Emory University School of Medicine, says, “The human eye is limited. We only rely on what we see in front of us—the pathologic diagnosis and the scans.”
A lot has changed in a decade
Today, Winship is home to a host of cutting-edge research in artificial intelligence (AI) that has the potential to drastically shift how physicians approach cancer care, from diagnosis to treatment to long-term survivorship. Powerful programs are available to support human eyes, able to recognize patterns, connections and warning signs far below our sensory thresholds.
Recalling his patient with tonsil cancer, Saba says, “I think perhaps AI could have detected that this is not your regular-behaving HPV-related disease. If we had known about this outcome, we would have treated these patients differently from the beginning.”
From imagination to (virtual) reality
From the realm of science fiction to a sudden, very real reality, artificial intelligence is here—and it’s rapidly shifting the fundamentals of how we live and work.
From handy uses like digital personal assistants to more insidious applications like deepfakes—realistic but false digital recreations of celebrities saying things they never really said, or fabricated news footage that spreads misinformation—AI harnesses machine learning algorithms and neural networks to teach computers how to perform what was previously inconceivable. AI creates in ways that were once open only to human minds, and on a scale far vaster than our brains can conceptualize unaided.
This revolution reaches into scientific discovery at Winship, where researchers are increasingly using the tools of AI to reimagine how doctors diagnose and treat cancer. In this setting, the AI-related work at Winship happening across fields and types of cancers has the potential to help turn widespread precision medicine from a pipe dream into the new standard of care. It can help to increase the efficiency and accuracy of radiology work, and even forge more equity in a field with stubbornly persistent racial disparities in patient outcomes.
“As we get more and more information, there are only so many crunches that we can do as humans,” says Sunil Badve, MD, a member of Winship’s Cell and Molecular Biology Research Program and professor and vice chair of pathology cancer programs in the Department of Pathology and Laboratory Medicine of Emory University School of Medicine. “That’s when computers come in and start crunching all these different relationships. Out of the whole haystack, all these needles are pulled out and brought together, and now we can have a better understanding of what’s going on.”
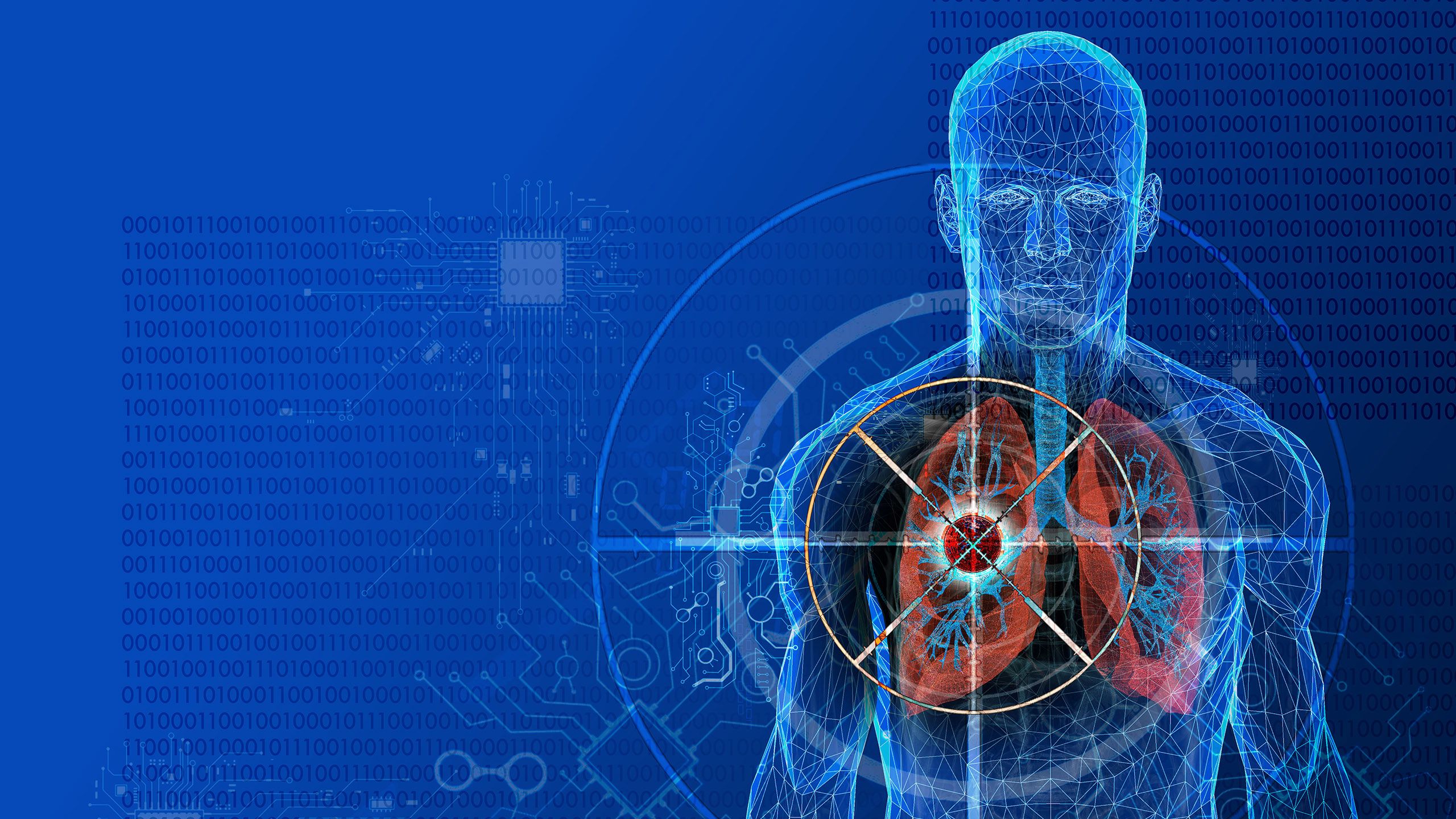
Anant Madabhushi, PhD, a member of Winship’s Cancer Immunology Research Program, is deeply and publicly involved in Emory’s AI work. A professor in the Wallace H. Coulter Department of Biomedical Engineering, Madabhushi holds secondary appointments in the Departments of Radiology and Imaging Sciences, Biomedical Informatics (BMI) and Pathology. Madabhushi collaborates with faculty across numerous disciplines to discover the potential of AI in cancer detection, treatment and survivorship. He is also the executive director of the Emory Empathetic AI for Health Institute (AI.Health), which launched in November 2023.
AI.Health, under the umbrella of Emory’s campuswide AI.Humanity Initiative, seeks to harness AI to improve cancer detection, risk and treatment response predictions. And it seeks to do so in studies that incorporate AI technology from the start. Utilizing AI in research from the start represents a push forward from the current status quo, where most work is retrospective, since algorithms need to learn on big sets of pre-existing data. Madabhushi says this approach will provide tools to better stratify risk before treatment and to monitor the patient’s response early on.
AI-related research at Winship could ultimately change cancer medicine by offering the option of less extreme regimens with fewer side effects for cancers less likely to recur. “Our goal is not just to develop algorithms from a diagnostic perspective, but also to be able to prognosticate disease outcome and predict therapeutic response,” says Madabhushi.
Anant Madabhushi, PhD, executive director, Emory Empathetic AI for Health Institute
Anant Madabhushi, PhD, executive director, Emory Empathetic AI for Health Institute
Sunil S. Badve, MD
Sunil S. Badve, MD
Abeed Sarker, MD
Abeed Sarker, MD
Mylin A. Torres, MD
Mylin A. Torres, MD
Signals in the noise
In 2022, Madabhushi and his co-authors published a paper in the journal Science demonstrating, for the first time, that the appearance of the vasculature (arrangement of blood vessels) around a lung tumor is indicative of whether or not the cancer is likely to spread. The vessels feeding tumors are more “twisted” in cases where they are likelier to be treatment resistant. In more responsive tumors, the vasculature appeared more orderly. AI could recognize this patterning because of its ability to detect subtle patterns within large, unwieldy data sets.
The new knowledge could potentially help doctors see how treatments are working before standard indicators, like change in tumor size, are visible. “AI is now prizing out subtle hallmarks that might give us earlier evidence of treatment response or lack thereof,” Madabhushi says.
AI’s ability to analyze huge quantities of data and detect patterns that human eyes can’t discern unaided is one way the technology may completely change the course of cancer diagnoses and treatments, alerting doctors to disease indicators yet to be discovered.
“What we are trying to do in cancer research is forecast the causality—the likelihood—of therapeutic benefit, and ultimately whether the patient is going to survive,” says Badve. He has been collaborating with Madabhushi since 2015 on using AI to predict which cases of DCIS, or “stage 0” breast cancer, will progress to invasive disease. “Survival is the goal,” he says. “Can we predict it based on whatever parameters we have?”
Badve’s own work looks at breast cancer using multi-omics—or multiple biological systems, such as the microbiome and the genome—to discover patterns and relationships. “As you increase the dimensionality of the data, you’re going to be able to understand more facets,” he says.
As we get more and more information, there are only so many crunches that we can do as humans.
The increased information also allows algorithms to start identifying things through increasingly smaller fragments. “If we are familiar with somebody, we can see their back or the way they walk and know who it is,” says Badve. Similarly, we recognize known faces while iPhone facial recognition “knows” the same face by analyzing collections of data points. The idea for AI, Badve says, is that computers “get better and better at recognizing things even without 100% of the image being there.”
AI-based pattern detection reaches beyond what’s happening inside a patient’s body. Abeed Sarker, PhD, a member of Winship’s Cancer Prevention and Control Research Program and an associate professor in the Department of Biomedical Informatics at Emory University School of Medicine, uses machine learning to scrape social media datasets to learn what people discuss in community and how it differs from what they say to physicians. In his collaborations with Winship, Sarker has pulled information from patients with breast cancer tweeting about their experiences on why many of them—especially younger patients—don’t adhere to some medical recommendations. These are the type of data that are rarely gathered by physicians and, if they are, are unlikely to be recorded in patient electronic health records.
Sarker wondered whether there are other sources of information that can be used to identify why people are not adhering. He and collaborators Mylin Torres, MD, a breast cancer expert and researcher who co-leads Winship’s Cancer Prevention and Control Research Program, and Mayo Clinic AI scientist Imon Banerjee, PhD, built an algorithm and a data set of patients with cancer tweeting about their experiences.
“We saw a lot of breast cancer survivors on whom the treatments are working fine, but it’s impacting their everyday lives,” Sarker says. “That is specifically a problem with younger people, because they may want to keep their social lives active, and these cancer treatments are not allowing them to.”
The team earlier presented a conference poster that explored the feasibility of combining electronic health records with social media posts. Most Winship patients approached for that research were happy to provide access to both platforms to seek new insights.
“These language models identify patterns in language that are generalizable and that can detect information such as disclosure of breast cancer status, disclosure of side effects, disclosure of sentiment,” Sarker says. “One person saying something does not really make a signal. If there are hundreds of people associating negative sentiment with a medication, that is a signal.”
Diagnostic precision
Another major focus of Winship’s research at the intersection of AI and cancer involves using the technology to improve the precision of diagnoses and treatment planning. This helps to better individualize treatments for each patient while saving radiologists time and improving their accuracy. Which is to say, researchers are using AI in cancer diagnosis to realize the potential of precision medicine.
“We want to use advanced imaging to more accurately define the target,” says Xiaofeng Yang, PhD, DABR, a member of Winship’s Discovery and Developmental Therapeutics Research Program and an associate professor and vice chair for medical physics research in the Department of Radiation Oncology of Emory University School of Medicine. Yang’s work integrates machine learning with MRI to improve predictions of tumor recurrence and decrease the amount of time it takes to read the images needed to diagnose and create treatment plans. For example, he says, it takes doctors about two hours to manually map out radiation pathways for head and neck cancer treatment. With AI assistance, that work can get done in under half an hour, saving valuable time and resources.
Yang’s research also looks at incorporating AI into medical imaging. This can help personalize treatments by, for example, using stronger protocols for cancers that are more likely to recur, or identifying tumor borders with increased precision to allow for more targeted radiation. This lessens the chance of severe side effects that occur with larger tumor borders.
Besides personalizing standard treatments, this AI-aided pattern recognition can help realize the potential of personalized (precision) medicine, which creates protocols specifically tailored to a patient’s own biology. It’s long been considered, but has yet to become, the gold standard toward which many cancer treatments are heading.
“The response rates for immunotherapy are something like 20% to 25%,” says Madabhushi, referring to the type of personalized medicine that harnesses patients’ own immune systems to help eradicate disease. He says that using AI in pattern recognition before treatment helps to predict which patients will respond or not, again helping to hone precision medicine’s potential. AI can then help radiologists track whether treatments are working.
“People are notoriously bad at measuring things,” says Elizabeth Krupinski, PhD, a member of Winship’s Cancer Prevention and Control Research Program and professor and vice chair for research in the Department of Radiology and Imaging Sciences at Emory University School of Medicine. “People make mistakes. It’s very hard sometimes to measure tumor changes as a function of growth.” She adds, “Computers are far more consistent at the very least in what they measure and how they measure things.”
Krupinski, an experimental psychologist, looks at how radiologists incorporate AI into their workflows, and how they can do so in ways most likely to increase diagnostic efficiency and decrease errors.
AI can be presented in a number of ways. “There are heat maps, probability maps and sometimes there are little arrows pointing or segmentation,” Krupinski says. “You can give a lot of different kinds of information to somebody and you have three choices: it can have zero impact, it can have a positive impact or it can have a negative impact.” She assesses impacts through eye gaze and medical decision-making studies, giving radiologists AI tools and watching how they use them to see how they might do so more effectively. “If I can help radiologists decrease those error rates—to help them improve their performance and diagnose the images more accurately—that’s where I see my role,” she says.
Fellow Winship Cancer Prevention and Control researcher Judy Wawira Gichoya, MD, MS, associate professor in the Department of Radiology and Imaging Sciences at Emory University School of Medicine, has found that using AI to weed out malignancy currently decreases radiologists’ accuracy, because they don’t tend to double-check the images that AI labels benign as closely.
Learning how to work with AI’s capabilities instead of against it could be a game-changer. Technology that helps radiologists flag scans with a high likelihood of malignancy has the potential of improving both speed and accuracy, while lightening the load on overworked doctors.
Radiologists review upwards of hundreds of images daily, in long shifts that increase the chances of error. Some 54% of radiologists suffer from burnout, according to a 2022 Medscape report. Imaging Technology News called this a “crisis,” because “there are just not enough of us to get the work done.”
Krupinski says things are going to change “in a pretty significant way.” She cited the example of using mammograms to diagnose the presence of breast cancers. “The frequency of finding cancers is relatively low,” she says. “If an AI system could triage cases with a very high degree of certainty, finding cases that are truly negative, it would dramatically change the way mammographers read images. And it’s going to give them more time to look at the images where the AI thinks something is going on.”
Xiaofeng Yang, PhD, DABR
Xiaofeng Yang, PhD, DABR
Elizabeth Krupinski, PhD
Elizabeth Krupinski, PhD
Judy Wawira Gichoya, MD, MS
Judy Wawira Gichoya, MD, MS
Increasing equity
Just like virtually every other metric of health and well-being in the United States, cancer diagnosis and survival rates contain race-based disparities. Although white people are most likely to be diagnosed with cancer in this country, Black and Indigenous people are more likely to die from it. To cite just one specific example, Black women are diagnosed with breast cancer 4% less frequently than white women are, but have a 40% higher mortality rate.
While systemic racism has produced a multitude of factors that create and perpetuate discrepancies, AI has the potential to help diminish them—but only if the algorithms are trained on data sets that include sufficient representation from all races and ethnicities.
Like most medical research, existing AI algorithms are primarily trained on data sets made up of disproportionately white patients. The implications are huge: if diseases present differently across races, and machines can only accurately predict patterns as they appear in one of them, deploying AI more widely will only increase health care disparities as algorithms reinforce biased recognition patterns.
“The goal is that we have to make sure that these tools really work across a plurality of populations,” says Madabhushi.
Winship researchers repeatedly note that one strength of the institute’s research program is that it treats a remarkably diverse patient population, allowing their AI to train on data sets more representative of the global population.
Madabhushi says it’s difficult to interpret the where, how and why of AI’s algorithms. “While it can generate some pretty amazing, unexpected predictions or results,” he says, “the challenge is that it is very unclear as to what the basis or the bedrock of those predictions is.” This means that if the system ends up being biased, it’s basically impossible to understand where it went offtrack.
The demographic advantage of being located in Atlanta and serving the area’s diverse patients is prioritized by many of Winship’s AI researchers, who stress that building equity into new technologies is essential. “I don’t think there is any place like Emory Healthcare and Winship that has such diversity in their cohorts,” Sarker says. “I think it’s very unique in the United States.”
Gichoya says her work as a member of Winship’s Cancer Prevention and Control Research Program, and a core part of Empathi, is about “promoting health equity using data science.” She compiles diverse data sets with multiple types of imaging—such as pathology and radiology pictures—“to leverage images that are routinely acquired to see what else they can tell us about the patient,” and to expand researcher access to these sorts of resources. She says AI’s scaled pattern recognition ability can identify the “hidden signals” in images that human eyes wouldn’t identify as significant. This presents many opportunities, says Gichoya, such as her and her collaborators’ finding that it’s possible to identify a patient’s race with high accuracy using AI to look at patient imaging.
This and other indicators can give information about “how fast you’re aging, what sort of area you live in, your sex,” Gichoya says. It can allow physicians to learn about how people live, what their prognosis might be and how to tailor treatments accordingly. Badve notes that combining a multidimensional analysis of tumors with patient demographics might allow for more accurate predictions of how the cancer will behave, again leading to more personalized treatments.
The research focus on realizing the great potential—and avoiding the pitfalls—of AI may well have broad impacts on patient health as Winship methods, technologies and mentees proliferate. But all the innovation is ultimately aimed at one thing: helping the next patient facing a terrifying cancer diagnosis get the best possible chance at recovery and a long, symptom-free survival.
“I think it has a great potential to change things rapidly for us,” Saba says, “to hopefully lead to faster discoveries and better translation to improving care for patients.”
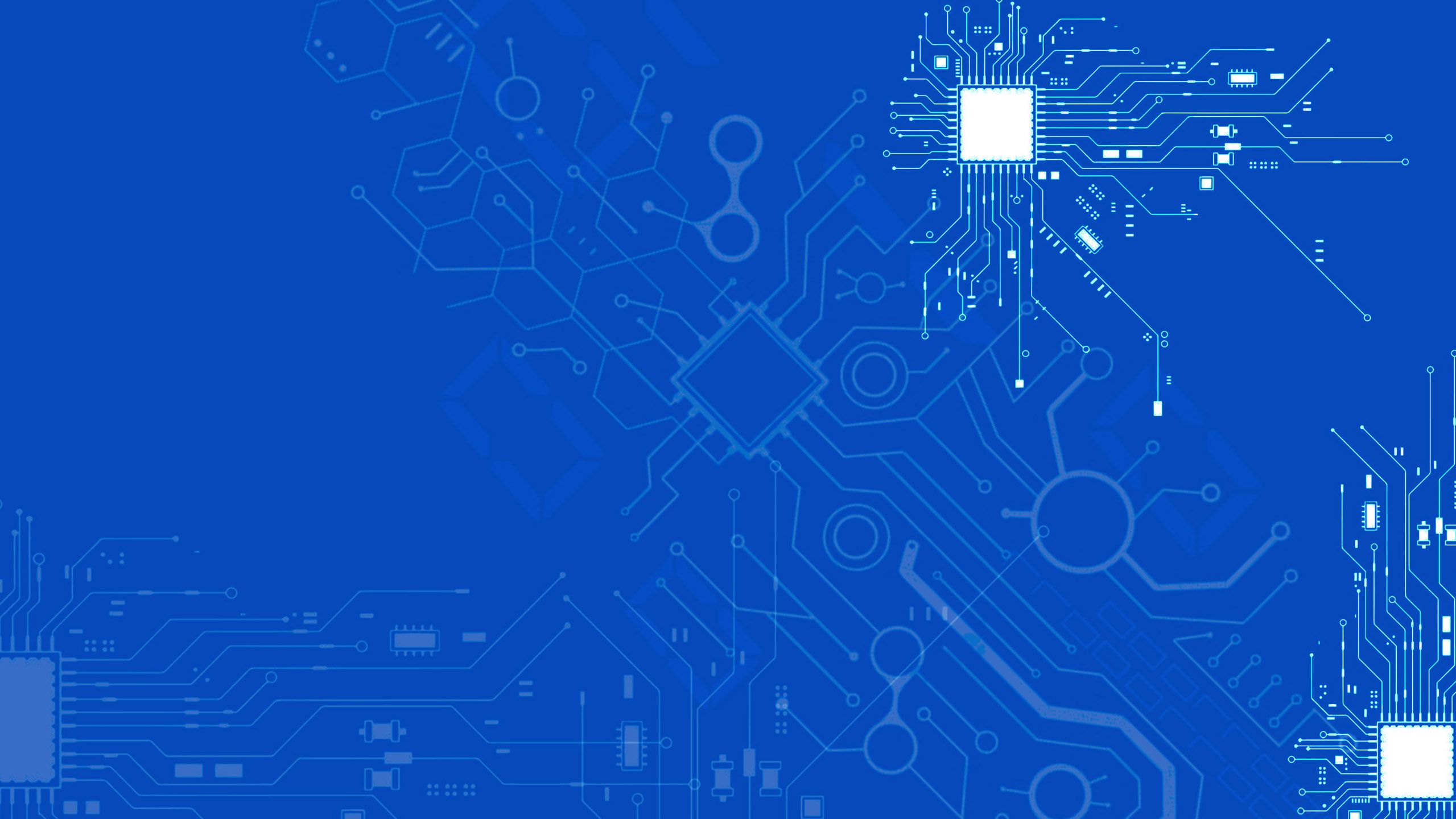
Care tailored to your needs
Care for patients with cancer at Winship includes leading cancer specialists collaborating across disciplines to tailor treatment plans to each patient’s needs; innovative therapies and clinical trials; comprehensive patient and family support services; and a care experience aimed at easing the burden of cancer.
More from Winship Cancer Institute
Winship researcher leads Emory's new AI for health institute
The first institute of its kind in Georgia, Emory AI.Health, led by Winship member Anant Madabhushi, PhD, fosters the development of accessible, cost-effective and equitable AI tools including methods for improving cancer diagnostics and treatment. Collaborating with multidisciplinary experts, the institute aims to leverage AI to save and improve lives, making cancer care more proactive and personalized.
Cancer Immunology Research at Winship
The Cancer Immunology Research Program at Winship Cancer Institute of Emory University conducts research aimed at preventing and treating cancer through harnessing the immune system. The program aims to identify the cellular and molecular mechanisms regulating antitumor immunity and testing new immunologic approaches for cancer treatment and prevention including the use of artificial intelligence.
Winship Magazine Spring 2024
The Spring 2024 issue of Winship Magazine features how artificial intelligence is changing cancer medicine, highlights a patient story of Keith finding his "warrior mode" , and describes how Winship is contributing to the Cancer Moonshot initiative, and much more.
Did you like this article? Read more features in Winship Magazine.